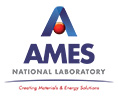
Ames National Laboratory
Coordinator: Peng XuReview abstracts for current and past practicum experiences at Ames National Lab >>
Ames National Laboratory proposes a new emerging core capability in Computational Science, based on leading research in exascale computing and computationally-assisted scientific discovery. Through years of developing scientific software, particularly our 10-year involvement leading software development for two Exascale Computing Project (ECP) applications, we have a core set of researchers and exascale-capable expertise. We lead quantum computing efforts through BES-MSE funding and through the Superconducting Quantum Materials and Systems Center, led by Fermi National Laboratory.
Exascale computing for chemical and molecular sciences: The Laboratory led efforts in development of two exascale-computing capable computational chemistry codes with efficient and portable implementations on heterogeneous architectures: the General Atomic and Molecular Electronic Structure System (GAMESS), and NWChemEx. These codes support a broad range of chemistry research important to DOE Biological and Environmental Research and DOE Basic Energy Sciences on computing systems that range from petascale servers to exascale computers. Ames Lab has a core set of researchers continuing to work to develop these packages, and to demonstrate their value for chemical and molecular sciences.
Accelerated Optimization and Development of Molecular Synthesis: The Laboratory has pioneered the development of AI-driven laboratory scale optimization and automation algorithms and software, Rxn Rover. Rxn Rover can automate laboratory hardware to perform unassisted process optimization and achieve data-enabled prediction of process conditions to accelerate molecular synthesis. A large range of algorithms have been used and developed to facilitate the fastest time to solution.
Quantum computing for predicting correlated material behavior: The Laboratory leads efforts seeking to achieve practical quantum advantage by applying quantum algorithms to calculate properties of correlated multi-orbital quantum materials. The application of adaptive, variational quantum algorithms may provide a path to practical quantum advantage with an appropriate combination of problem formulation, algorithm, and hardware development.
AI-accelerated material and chemical discovery: Ames Lab is developing and applying a number of AI-accelerated approaches for materials discovery, ranging from physics-informed prediction of magnetic properties of novel compounds, to ML-accelerated genetic algorithm prediction of novel crystal structures in unexplored compositional space. We are utilizing AI-based molecular and protein design for rare earth separations, and developing new UQ approaches for integrating limited experimental data with simulations to optimize materials processing for efficient manufacturing.