Fellow Contributes to Machine-Learning for Ion Acceleration Research
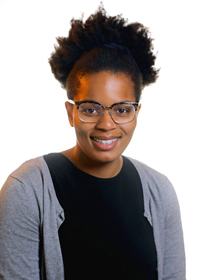
A Department of Energy National Nuclear Security Administration Laboratory Residency Graduate Fellowship (DOE NNSA LRGF) recipient is part of a team applying machine learning to study laser-driven ion acceleration.
The model that Raspberry Simpson and colleagues from Lawrence Livermore National Laboratory (LLNL) produced can help researchers explore combinations of parameters that affect this high-energy physical process. The machine-learning model can serve as a surrogate for simulations and experiments. It was the first time scientists used machine learning to study laser-plasma acceleration.
The project, published in the journal Physics of Plasma, “primarily serves as a simple demonstration of how we can use machine-learning techniques such as neural networks to augment the tools we already have,” primary author Blagoje Djordjević, an LLNL postdoctoral researcher, said in a lab release. Computationally expensive simulations “will remain a necessary aspect of our work, but with even a simple network we are able to train a surrogate model” that can explore interesting experimental conditions.
Simpson is a plasma physics doctoral student at the Massachusetts Institute of Technology. She worked in residence at the lab in 2019 and 2020. Fellows must serve a minimum of two residencies at one or more of four NNSA facilities, giving them access to unique facilities and expertise.
The machine-learning model focuses on high-intensity short-pulse laser-plasma acceleration, specifically for propelling ions from solid targets. The laser pulses interact with the target, pushing electrons that can accelerate protons or heavy ions or produce bright X-rays. But the laser-pulse shape and duration can vary widely. Researchers must use experiments and simulations to study combinations of parameters and their effects on acceleration.
The team conducted more than a thousand laser-plasma acceleration simulations and extracted ion energy, electron temperature and other physical parameters from them. Those data were fed into the neural network program, which trained it to act as a surrogate model that rapidly explored parameter space and mapped the dependency of ion energy on laser intensity and pulse duration over orders of magnitude.
“Using a sparse but broad dataset of simulations, we were able to train a neural network to reliably reproduce the trained results as well as generate results for unsampled regions of parameter space with reasonable confidence,” Djordjević said. “This resulted in a surrogate model, which we used to rapidly explore regions of interest.”